How Can Data Enable Tackling the Climate Crisis and Rising Fuel Poverty Through an Efficient Rollout of Retrofit?
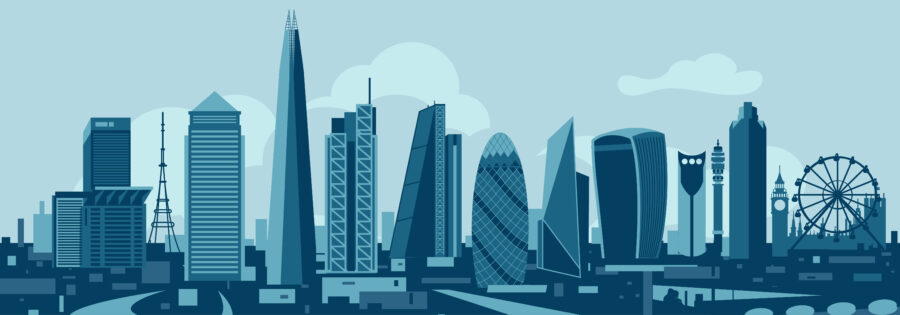
This article forms part of our Data for Policy series. As part of IPPO and UCL’s Department of Information Studies’ Building Local Data Capabilities project, five Data for Policy Fellows are currently embedded in partner local government bodies across the United Kingdom and will write about their experiences and insights on the challenges of using data in policymaking.
Maria Wood
The London Building Stock Model
The Greater London Authority (GLA) is London’s regional governance body, comprising the Mayor and the London Assembly. It administers the city’s transport, housing, policing, fire, and economic strategy and development. In 2017, as part of the Mayor’s Fuel Poverty Action Plan, the GLA partnered with UCL to produce an open-source model of all domestic and non-domestic buildings in London named the London Building Stock Model (LBSM). This model synthesised and visualised the energy performance (mostly in the form of Energy Performance (EPC) and Display Energy (DEC) Certificates data), building height, building type, floor area, and non-domestic activity for every building in London as a resource for developers, community organisations, and more.
Now, the GLA is developing version two of the LBSM. A diverse range of experts, including energy experts in the GLA Energy Team and data scientists, data engineers, GIS specialists, and data visualisation experts in the City Data team are working together to upgrade and update the LBSM such that it can be utilised effectively by stakeholders including London boroughs, developers, retrofit companies moving forward. The LBSM v2 will focus on domestic buildings in London, pointing to the household fuel poverty issue at the core of the work’s initial concept. It will also be dynamically updated with new data to ensure that it is always up-to-date, an improvement on version 1, which gave a static image based on 2017 data.
Opportunities of this City-Wide Dataset
During my placement at the GLA facilitated by IPPO in collaboration with UCL’s Dept Information Studies, I am working with the City Data team within the City Intelligence Unit (CIU) and the Energy team to validate the outputs of the new London Building Stock Model (LBSM). Many building properties modelled in version 2 are relevant for various applications. Indeed, the combination of energy efficiency data (energy consumption and EPC data as well as type, roof and wall insulation, age, glazing, and heating system) with other data such as deprivation, income, tenure, and other socio-demographic information means that detailed insights into opportunities for retrofit projects are numerous.
There are already specific applications of the LBSM v2 that will benefit both residents and local authorities. There are two sub-regional Local Area Energy Plans (LAEPs) underway for North and South London, which will employ the building model’s outputs to identify the most effective decarbonisation pathways. For example, property-level heating systems and energy efficiency data can be used to identify priority areas within the sub-regions for retrofit, such as wall or roof insulation and heat pump installation. Addressing these priority areas first will ensure that the “easy wins” are completed, where maximum benefits are delivered to residents rapidly at the lowest cost.
Another example of the LBSM v2’s use includes new national waste collection schemes. The LBSM v2’s property type data will facilitate the planning of waste collection routes in the city; apartment blocks, detached homes, and flats above retail units all have unique waste management needs.
There are also many other applications of the model already emerging. For example, the LBSM’s number of bedrooms data will enable more accurate estimations of the city’s current and, therefore, projected population, which is used in planning decisions across London. Utility and retrofit companies, as well as solar panel estates, will be able to use the data to effectively and efficiently plan future works for maintenance, development, and upgrades.
Finally, a complete, detailed, and publicly available city-wide dataset ensures that individual boroughs are able to use their data capabilities more efficiently, saving capacity for locally-specific work. Since data literacy and capabilities are varied across the London local authorities, this work will provide a baseline from which all 33 local authorities can build. For example, it will facilitate the streamlining of projects that are geographically close to one another spanning multiple boroughs, reducing not only costs but also reducing the need for specific data capabilities within the local teams.
Challenges: Missingness, Quality Assurance and Geographic Inconsistencies
This project is unveiling a variety of information challenges. There is a distinct amount of missing data in some of the key datasets used in building the LBSM v2; though the model’s purpose is, by definition, to fill these gaps, especially patchy input data can be a challenge. For example, many properties have missing or outdated EPC data since EPCs are only conducted when homes are sold or rented. Moreover, the EPC rating process relies on predictions rather than measurements, resulting in often inaccurate data. These issues can be mitigated by combining multiple independent datasets to cross-reference outputs, such as gas and electricity meter readings, to corroborate energy consumption estimates. Furthermore, engaging with social landlords and housing associations to share the detailed building data that they collect and own for their buildings will enable more accurate predictions to be made overall.
Similarly, quality assurance of the LBSM’s outputs can present challenges. One way that this can be done is by comparing the outputs to reliable sources of aggregate data, such as the English Housing Survey or at the building level for specific modelled fields. Validating the outputs early in the process has proven to be a key step in ensuring the output is robust.Another overarching issue faced by the GLA in this work is the inconsistency between datasets that are often found; each dataset follows different conventions on both geographical aggregation and specific building labelling. For example, while some Ordnance Survey geographical data is recorded at the building level (using TOIDs), some is at the property level (using UPRNs) while others, such as Census data, are given at different geographical aggregations such as output areas. Some of these issues have been overcome through address matching or spatial aggregation, though others have proven harder to unpick.
How to Ensure Your Dataset Delivers the High Quality Insights for Policymaking
Since policies based on data insights require representative and accurate data, it is essential to ensure high-quality data at all stages of the modelling process. For input data, this looks like using granular, high-coverage data from reliable sources, such as the Census, Ordnance Survey, and utility companies. Validating the outputs is also essential: we have found that cross-referencing different datasets is a highly valuable step in the validation process.
Engagement with local stakeholders is an important aspect of utilising data for policymaking. Not only can data be flawed or missing, but it often does not tell the whole story. As such, combining raw data with more nuanced views and specific insights from local stakeholders, such as social landlords, housing associations, or utilities, will enable a more holistic and true-to-life decision-making process.
Overall, developing a city-wide building stock model proves to be a hugely important asset for a wide range of policy applications. The development of a similar model would likely be useful for other combined authorities. Much of the input data used in the LBSM v2 is national data, not limited to the London geography, meaning this could be a feasible goal. Indeed, the expanding development of LAEPs across the country will be benefited by these housing stock models in the same way that the London sub-regional LAEPs are employing the LBSM v2 outputs. Similarly, the above-mentioned UK waste disposal scheme overhaul will be applied nation-wide, giving another direct application of these building models.
Maria is a Data for Policy Fellow at UCL’s Dept of Information Studies and first-year PhD student at UCL’s Centre for Advanced Spatial Analysis where she is researching transport justice and how to leverage emerging demand-side technologies to deliver a just transition. Prior to this, she gained her master’s in physics and spent two years working as an energy consultant, specialising in whole systems energy modelling.