The Synthesis Gap: reducing the imbalance between advice and absorption in handling big challenges, from pandemics to net zero
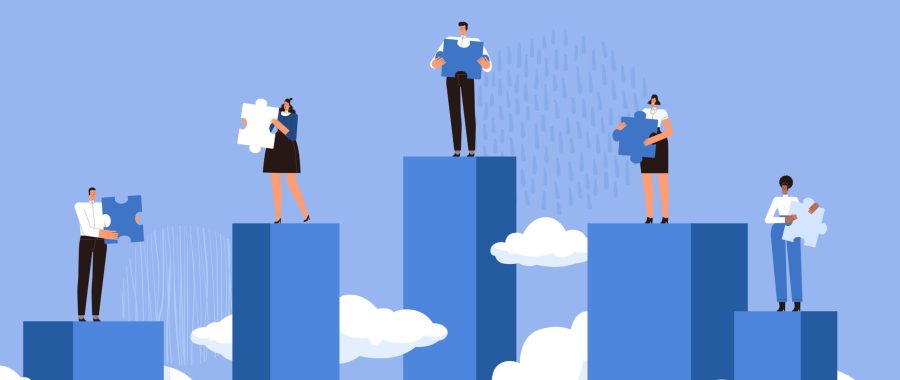
Good synthesis results in higher-value options and actions. This paper focuses on how governments should synthesise inputs from many disciplines and sources to make decisions – whether during a pandemic or to guide a major strategy. But the issue of what makes a synthesis good is much broader
Geoff Mulgan
How should different kinds of data, insights, knowledge and interests be integrated to guide understanding or action – or to put it simply, how should we synthesise? (1)
This paper draws on the first year of work by the International Public Policy Observatory (IPPO), collaborating with researchers and policymakers on how to respond to the many challenges of COVID-19, from education and care to mental health and housing. It also draws on decades working with governments around the world to improve strategic thinking and action.
Primarily, the paper focuses on how governments should synthesise inputs from many disciplines and sources to make decisions – whether during a pandemic or to guide a major strategy (2). But the issue of what makes a synthesis good is much broader, relevant to anyone interested in data and knowledge, or in the future of artificial and collective intelligence – which need methods that can integrate multiple voices or inputs in a coherent way (and which suffer from a parallel synthesis gap). The issues are also relevant to research teams seeking an understanding of complex phenomena (e.g. gang crime or public behaviour in recycling), to public inquiries making sense of thousands of inputs, or to cities seeking a shared vision of the future.
The paper suggests both theoretical perspectives and practical ideas about how to do synthesis better. It was prompted by a concern that the absorptive and synthesising capacity of governments is often weak, and in some cases may have declined. This means that even when there is high-quality advice and analysis, and skilled knowledge brokerage, this does not lead to optimum actions. The US and UK are striking examples: endowed with the world’s most admired universities, and deep pools of expertise, but performing relatively poorly through the COVID-19 pandemic, and lacking strong capability for synthesis.
The paper argues for more conscious attention to synthesis – both in universities and in organisations, such as governments, that are responsible for whole systems. It shows that synthesis can follow a series of stages which can be mapped logically, and that the skills for doing this well can be learned and embedded in teams.
Good synthesis results in higher-value options and actions. That it is often done badly, or not at all, matters.
The problem: an imbalance between inputs and digestion?
All over the world governments use advisory mechanisms – often bringing together scientists and social scientists to feed into the government machine, offering insights and evidence. Sometimes these are orchestrated by people with formal advisory roles such as Chief Scientific Advisers, Chief Economists, Chief Medical Officers and so on. More advice is in principle a good thing. However, governments face fundamental challenges in handling advice.
The first is to ensure that the right kinds of knowledge are drawn on, which may include all on the following list (each of which has its own professions, networks and ways of thinking):
- Statistical knowledge (for example of unemployment rises in the crisis)
- Policy knowledge (for example, on what works in stimulus packages)
- Scientific knowledge (for example, of antibody testing)
- Disciplinary knowledge (for example, from sociology or psychology on patterns of community cohesion)
- Professional knowledge (for example, on treatment options)
- Public opinion (for example quantitative poll data and qualitative data)
- Practitioner views and insights (for example, police experience in handling breaches of the new rules)
- Political knowledge (for example, on when parliament might revolt)
- Legal knowledge (for example, on what actions might be subject to judicial review or breach Human Rights Conventions)
- Implementation knowledge (for example, understanding the capabilities of different parts of government to perform different tasks)
- Economic knowledge (for example, on which sectors are likely to contract most)
- ‘Classic’ intelligence (for example on how global organised crime might be exploiting the crisis)
- Ethical knowledge about what’s right (for example on vaccinating children who may have relatively little risk from a disease)
- Technical and engineering knowledge (for example on how to design an effective tracing system or build a new high speed rail line)
- Futures knowledge (foresight, simulations and scenarios, for example about the recovery of city centres)
- Knowledge from lived experience (the testimony and experiences of citizens, usually shared as stories, for example about experiences of the pandemic)
There are many other ways of constructing or structuring such a list. But, however it is done, four crucial points follow from any recognition of the diversity of types of knowledge that are relevant to decision-making in governments.
- First, there is no obvious hierarchy or meta-theory to show why some types of knowledge might matter more than others. Leading figures in particular fields may feel that it is obvious why their knowledge is superior to other types of knowledge. But it is impossible for them to prove this convincingly. Moreover, the status and influence of these different sources of knowledge may correspond only loosely to what is needed at any particular time (the UK system, for example, often does better in mobilising scientific knowledge than more practical engineering knowledge, such as on how to handle data during a crisis – as indicated in this recent piece by past and present Scientific Advisers (3)). In democracies, political knowledge (and interests) can sometimes trump other kinds of knowledge – but in practice its legitimacy depends on acknowledging the claims of competing forms of knowledge (4).
- Second, there are no formal models or heuristics to show which kinds of knowledge, and which models or frameworks, are relevant for which tasks and when. There are many methods for summarising or linking different kinds of knowledge, using knowledge graphs and other tools. But the ability to know what to apply and when depends on a kind of wisdom that can only be gained through some familiarity with these different kinds of knowledge and their application, and through experience.
- Third, values are every bit as important as knowledge to framing decisions, and synthesis is bound to be as much an ethical and political task as a purely rational one (5). Values shape what issues are thought to matter; they shape what perspectives and voices are listened to; and they shape how different options for action are judged. Sometimes they are ‘added in’ through hunch and intuition but they can be mapped and interpreted more systematically than ever (6).
- Fourth, any decision-maker needs to synthesise or integrate the often-contradictory signals coming from the different kinds of knowledge listed above, and to connect them to values, beliefs and public aspirations. This isn’t helped by the fact that each of the fields of knowledge has its own jargons and languages, and usually struggles to understand the others. Anyone tasked with judging which to apply and when, and how to combine them, has to be unusually multi-lingual.
It is sometimes assumed that this job (which goes well beyond knowledge synthesis) will be done by politicians, helped by political advisers – but they rarely have the time or skills to do it well. Sometimes it is assumed it will be done by senior civil servants – but again, they may or may not have the skills to do it well (and are likely to be much more confident dealing with issues of law and economics than with data or science). Even the most sophisticated accounts of science advice and knowledge brokerage still present it as an input and support for decisions that are taken by others, leaving the crucial moments of decision as a kind of ‘black box’ (7).
The result is often an excess of advice and a deficit of synthetic capability. Later I suggest some possible answers – but first I take a step back to look at what synthesis actually is.
What is synthesis?
The ability to synthesise, and make sense of complex patterns, is an essential part of human intelligence. However, there is also a long philosophical tradition of thinking about synthesis which has often contrasted it with analysis. Where analysis meant ‘breaking something complex down into simple elements’ (8), synthesis does the opposite, bringing things together in a new way (9).
In philosophy, that meant going well beyond aggregation. Instead, synthesis was often understood as a way for conflicts to become resolved in argument at a higher level. Immanuel Kant wrote of ‘the action of putting different representations together with each other and comprehending their manifoldness in one cognition’ (10). The synthesis captures the truth more accurately than a mere description of parts. Hegel was even more influential with his idea of a progression from thesis and anti-thesis to synthesis, where the synthesis contains and also transcends the truth of both the thesis and the antithesis, rather as male and female come together to create a new human (11).
A parallel tradition can be found in the arts. Samuel Taylor Coleridge wrote of ‘esemplastic power’, the ability to shape disparate things into one, to take combinations and make a new whole (12). A similar point was made by the great educationalist John Dewey, who saw imagination as a tool for synthesis: ‘A way of seeing and feeling things as they compose an integral whole. It is the large and generous blending of interests at the point where the mind comes in contact with the world.’ (13)
Types of synthesis
There are many types of synthesis. Here is a rough taxonomy:
- Synthesise downwards – putting multiple things into a single metric (like money as standard measurement, which is what cost benefit analysis tries to do, or the use of QALYs as a single metric for judging health interventions). This could be called ‘synthetic dimensional reduction’. Further examples include making many ingredients into a soup, and other kinds of what could be called ‘synthetic averaging’. (14)
- Synthesise upwards – examples include a frame or theory that makes sense of multiple and otherwise confusing things (like evolutionary theory). Another example would be synthetic metrics – eg HDI, GDP or scorecards, which try to capture many dimensions of a phenomenon rather than being reductive. Synthetic materials that have superior properties to their parts are another example.
- Synthesise forwards – drawing on many inputs to decide on a course of action or strategy, such as a military campaign or a plan for mass vaccination. (As Richard Rumelt put it in his book on strategy: ‘Reducing the complexity and ambiguity in the situation, by exploiting the leverage inherent in concentrating efforts on a pivotal or decisive aspect of the situation.’)
- Synthesise backwards – making sense of historical patterns in a narrative that distinguishes the critical factors (we understand our lives backwards but are condemned to live them forwards).
- Synthesise with analogy – by using a valid analogy, making sense of disparate elements: e.g. seeing the earth as a single organism (Gaia), seeing a pandemic as like a war, or seeing the spread of an idea as like a virus.
- Synthesise through a heuristic – i.e. simple decision rules that can work most of the time (e.g. a monetary policy target, or an injunction to business start-ups to focus on cash).
- Mosaic syntheses with pairs or threes, or multiple models, as building blocks towards a whole.
Purposes for synthesis
A good synthesis enlarges and deepens possibility space – deepening how well we understand a phenomenon and so expanding what options are open to us. But the value of any synthesis depends on what it aims to achieve and for who. This will vary greatly depending on social, institutional and political contexts.
Some syntheses prioritise understanding – for example, those done by a research team or thinktank. Other syntheses prioritise action – for example, by a government or business. In each case, too, the synthesis may prioritise the present (including the need to act very fast in a crisis) or the future.
This table summarises some of these different purposes:
Purposes | PRESENT | FUTURE |
UNDERSTANDING | Present understanding
Explanatory power (of a current phenomenon, e.g. behaviour in a pandemic) or retrospective judgment on actions (e.g. handling a pandemic). | Future understanding
Insights that emerge in the future from the emergence of new disciplines, research methods, or (for example) from longitudinal surveys. |
ACTION | Present action
Decisions on actions to impose lockdowns or travel restrictions balancing health, economic and social factors. | Future action
Decisions to act that are justified by the potentially dynamic or cumulative nature of the results (e.g. exponential economic growth, or acting to prevent future risks). |
So, for example, a synthesis to guide actions in the heat of a crisis with imperfect and ambiguous information (a terror attack, pandemic outbreak or cyber-attack) will look very different from the kind of synthesis a public inquiry may do many years later.
Similarly, a synthesis of evidence on online learning will be different if its primary aim is to understand what happened to schoolchildren during the pandemic, or if its priority is to guide teachers as to what they should do. It will also be different if its aim is to contribute to cumulative knowledge in the future, or if its aim is to help decision-makers during the next pandemic.
At various points in the COVID crisis, suggestions were made that governments should seek to develop meta-models that could analyse the trade-offs between health and economic effects, using some measures of QALYs or wellbeing. This would be an example of a synthesis for present action (as far as I am aware, no government actually did this).
Understanding and action
Understanding often has to precede action and is a necessary condition for action (though, of course, we do many things unthinkingly). But the relationship between the two is quite complex.
Syntheses for understanding (such as syntheses of evidence) tend to be paradoxically both less complete and more complex than syntheses for action. They are less complete because typically they exclude crucial dimensions – for example, syntheses of technical options for carbon reduction tend to ignore the practical politics of implementation – how to persuade people to change their behaviours, accept new taxes and charges – yet these are vital for anyone needing to act.
On the other hand, such evidence syntheses are likely to be more complex than the actions that build on them. Indeed, actions are always simpler than the environments they are seeking to influence: we complicate to understand but simplify to act. (15)
The issues become even more complex with AI. The essence of machine learning is that it makes predictions in order to guide action, but doesn’t necessarily require understanding. The same may be true of complex models such as those for climate: it’s more important that they predict well than they necessarily understand why they make the predictions they do.
Seven steps of a synthesis process
We can generalise some ideas as to how synthesis should be done. In food, music, poetry and other fields, there are an infinite range of methods. But for understanding or acting on complex systems there are fewer, and any synthesis function is likely to use processes for:
- Mapping relevant factors, inputs, causation, models, relationships, ideas and attempting to put them into a common language.
- Ranking these inputs, models or insights in terms of explanatory, causal or predictive power.
- Attempting mergers or combinations (sub-syntheses).
- Clarifying trade-offs and complementarities.
- Clarifying knowledge and power, i.e. which causal links are well or badly understood, and which ones are amenable to power and influence. (16)
- Jumping to new concepts, frames, models or insights that use these inputs but transcend them.
- Finally, interrogating and assessing these new options and judging how much they create or destroy value. (17)
This is often a circling rather than a linear process. It involves trying out, exploring and interrogating on the way to a viable answer. It’s not a one-off exercise of the kind offered by multi-criteria analysis or cost benefit analysis (18). It may start with one set of models, discover these are inadequate, and then bring in others.
Values and ethics run through every stage (and can’t simply be added in as another ingredient in stages 1 and 2). They shape what questions to ask at the beginning, and the choices offered at the end.
The sixth stage invariably requires different methods – more lateral and visual – since the human brain cannot grasp systems through linear prose or equations alone. It often also involves alighting on a narrative: a framing story that makes sense of complex patterns.
In short, the point of these processes is to clarify how far different goals or pathways can be aligned or integrated in novel ways; when there are unavoidable trade-offs; and when outcomes are incommensurable or conflicting. They mirror the way individual brains move between analytical and more holistic thinking (19).
Some of these exercises happen within an institution, but others are negotiations between organisations (or countries), and these can have some similar processes. Negotiations can either synthesise downwards (either with dimensional reduction or averaging) or synthesise upwards. Some negotiations end up averaging – splitting the difference, for example. Others discover a new optimum which is mutually advantageous. The best negotiations, by connecting multiple elements, allow a jump to a future that is better for all the participants (an example from recent history is the Northern Ireland peace process).
- The work of NICE is a good example of evidence synthesis focused on action: it guides NHS commissioning decisions helped by a tool for synthesising downwards – assessing all treatments in terms of their likely impact on QALYs (20).
- The IPCC is a good example of institutionalised synthesis for understanding, at least of the first four stages (it has less authority to do the other three). It produces formal syntheses (most recently AR6); it makes use of multiple models and processes of deliberation, seeking a collective intelligence that is superior to any of its component parts, as well as preparing transformation and mitigation pathways (though one its problems – which mirrors governments’ use of tools such as cost benefit analysis – is that these often become overly rigid and fixed, and fail to adapt to shifts in the environment). But the IPCC doesn’t cover synthesis for action – i.e. orchestrating global knowledge on what works in relation to decarbonisation.
- Judicial inquiries are another method for synthesis (mainly synthesising backwards, i.e making sense of an event, as well as making recommendations for the future). (21)
The good examples take care not to see patterns too quickly or to jump to conclusions (a very common problem in every field, from medicine to policing). They also combine models and modes of thought – not just linear logic and models but visualisations and simulations, since we can often see complexity more easily than we can grasp it logically. (22)
Governments facing pandemics have had to do similar syntheses but at great speed. In the absence of capacity, methods or time to do sophisticated syntheses, they have had to rely on synthesising with heuristics – like the conclusion that there weren’t trade-offs between health and economics, because tackling the health issues was a precondition for economic revival; or the heuristic that tough early action was usually better than procrastination or waiting for more data; or the heuristic that almost any level of public debt was better than a COVID-induced recession. However, although some of these heuristics worked well for a time, none worked well through multiple phases of the pandemic. (23)
Recombination within synthesis
The steps set out earlier emphasise that synthesis is about more than assembling multiple elements together. Instead, through recombination, it aims to create something new. There are many examples in history of elements which were well known but whose combinations were not. Gunpowder is one: its example prompted the German polymath Georg Christoph Lichtenberg to extend the principle of trying out combinations. He wrote that ideas left in isolation miss out on their potential, and become locked within disciplines or frameworks. He recommended deliberately taking them out of their normal contexts: ‘One has to experiment with ideas.’
This is the argument for deliberate recombination, using randomness and chance to find new combinations and syntheses – most of which will be useless, but a few of which may be very useful (in the way that ideas such as contagion, explore-exploit trade-offs, or opportunity costs have turned out to be useful in many fields). Indeed, most effective processes for synthesis seem to both break things down into component elements (stages 1 and 2 in the process described earlier) and then combine them in new ways.
How should we judge a good or bad synthesis – and what is over-synthesis?
For a synthesis to be good, it has to create additional value: additional insights or options for action that are more useful and more valuable than simply aggregating or assembling the component parts.
As indicated earlier, we can distinguish between synthesis for understanding and synthesis for action. In relation to understanding, a good synthesis, like a good theory, generates more useful knowledge both in the present and the future. In relation to action, it helps to guide actions that in retrospect, and with the best available knowledge, look the best. A bad synthesis, by contrast, destroys more value than it creates: it simplifies in simplistic ways or blocks off potentially positive and useful options for action. It has a flattening effect.
This matters for practical decision-making because there is always a risk of over-synthesis as well as under-synthesis. The best response to a complex problem – even if it has a single cause, such as a pandemic – may be an assembly of multiple elements rather than a single approach. This is very clear in the case of COVID-19, which has required multiple responses in relation to:
- Testing and tracing;
- Vaccines;
- Economic support for households and businesses;
- Policing;
- Care homes;
- Education;
- Homelessness;
- Mental health.
The key point is that the best responses in each of these cases are relatively independent of each other, and should be. To over-synthesise them would have been inefficient. Instead, the key is to see where there are important linkages and interrelationships, or where decisions have much broader impacts (such as lockdowns) and focus on these: enough synthesis, but no more.
This is a familiar point in engineering and technology, which involves assemblies of multiple sub-systems; for example to make a car, a computer, a building or an aeroplane. The interfaces matter, of course. But there is no need for every component to follow a single logic – and attempts to reinvent everything from scratch in an integrated way are usually sub-optimal.
In his influential book The Sciences of the Artificial, Herbert Simon went a step further. He advocated a universal approach to problem-solving: precise specification of parameters in codifiable elements, and breaking any big problem down into “well-structured” sub-problems.
This can have some virtues but, in retrospect, Simon overstated the case. Not many important problems can be dealt with as he hoped, and his argument that we could dispense with judgment and experience as ‘woolly concepts’ misses the point that these are the only tools with which we can judge which models or methods to apply to which problems. Moreover, reductionism doesn’t work well for systemic tasks like the transition to a zero-carbon economy. In other words, we need both to focus on sub-problems when possible, and to return to focusing on the system as a whole when necessary.
Selectivity and ‘bounded’ synthesis
Any kind of synthesis involves selection. It has to exclude and downplay some things – considerations, specialised knowledge or established interests – while prioritising others. It has to involve judgments about where deeper integration adds value, and where it risks destroying it.
Time and resources will always be limited, and will constrain just how many different types of knowledge and insight can be attended to. In this sense, synthesis will always be ‘bounded’. The key issue is whether the synthesisers are open to the claims of other kinds of knowledge that may be more useful than the ones they are familiar with.
All models are wrong, but some are useful. The critical question is whether the net value, in terms of understanding or action, is greater in the end. That value will, therefore, depend on context and purpose. This is true of a government trying to address multiple deprivation or intersectionality, or one attempting to decarbonise an economy. Neither can ever be wholly comprehensive; instead, choices have to be made about the greatest harms or the greatest opportunities.
How much diversity?
A related question is how much diversity is essential for synthesis. Diversity – which can mean many things – is now widely recognised as vital for innovation and much else (24). Greater collective intelligence generally depends on using more diverse inputs, perspectives and ideas, and there will be many political and social reasons why this is also good. (25)
The value of a diversity of perspectives justifies the proliferation of advisory taskforces and committees in many disciplines to provide advice to governments. We should want the people who ultimately have to make decisions to be familiar with multiple perspectives, models and frameworks; there is lots of evidence to show that people overly steeped in a single discipline or a single world-view are less likely to understand how the world works or to predict well. (26)
In some fields, it is also now normal to involve the people most likely to be affected by policies in shaping them – for example, involving teenagers in shaping policies for future skills. But in other fields this is still very rare, and often crucial decisions are made in government with no one around the table with direct frontline experience of the issues being discussed.
But diversity doesn’t automatically lead to greater value for any of the participants without an effective method of synthesis. At worst it generates noise, or elements that cancel each other out – ‘dialogues of the deaf’.
This is a common challenge in meeting or consultation design, and in the operation of crowdsourcing sites such as Wikipedia. It is partly solved by designs and standards, and partly by creating specific roles for overseeing the work of synthesis – editors, judges, moderators, curators – whose task is to guide the process of combination, and to spot which ones add value and which ones don’t.
The job of creating coherence out of diversity also depends on meta-languages that can help people from different backgrounds understand each other’s concepts and viewpoints. Stories can be useful for this. So can logic. In the past, I used systems thinking as a technocratic meta-language to connect people with backgrounds in policy, data, engineering and social sciences, focusing them on causation and confidence: i.e. what causes what, and with what confidence can any particular pattern of causation be described? (27)
Who synthesises, and with what mindsets?
Who can synthesise or commission syntheses? The simplest answer is that synthesis is fractal and can be relevant at multiple levels. The most obvious commissioners of syntheses are peak powers – Presidents, Prime Ministers, Mayors and bodies such as the European Commission. Within some systems, there may be umbrella bodies with authority to guide the system. But any organisation can choose to contribute to more systemic and synthetic views.
Moreover, in many fields, there will be no obvious locus for synthetic work and an absence of leadership. In these contexts others have to stand in their shoes, but to do so in ways that address the limits of their legitimacy or capability. So, for example, if they come from philanthropy, they have to prioritise accountability; if they are a party politician, they have to find ways to involve the other side; if they are an academic, they need to prioritise also mobilising practical knowledge and citizen input.
In all cases, it is unlikely that any one individual will be capable of doing this work alone. Instead, it usually requires T-shaped teams which combine both specialised and generic knowledge, and skilled integrators with enough understanding of the components to piece them together. This essentially is what strategy teams are meant to do – work I have been heavily involved in for over 25 years (and it is welcome that there is revived interest in strategy within UK Government). Such teams need to straddle multiple fields and networks (28): my experience was that these teams ideally combine quite technical and analytical knowledge; direct experience (and tacit knowledge) of the frontline context in which the strategy would be implemented, and the practical challenges of implementation; engagement of beneficiaries; and, crucially, understanding of political and organisational environments. (29)
This requires distinctive mindsets, suitable for collaboration with others from very different backgrounds and interests. The greatest enemies of collaboration are distrust, arrogance and hubris. Without trust, people will tend to hide and hoard information. Those who believe that they are uniquely endowed with insights or abilities will tend to be poor collaborators and poor listeners. In highly collaborative environments, by contrast, people leave their egos and narrow interests at the door and commit to a larger common interest. They recognise the limitations of their own knowledge and perspective. This is where ethics, personality and style intersect, and where synthesis has a moral dimension beyond its technical components.
Technologies for synthesis
There is, as yet, no software that can synthesise for us: computation is much better at analysis than synthesis. But technologies can help, and we are in a fascinating period of experimentation to develop technologies that can support synthesis.
Our work at IPPO draws on the use of Microsoft Academic Graph by the UCL EPPI-Centre to help map meta-studies of evidence. Knowledge graphs are becoming a useful way to map fields of knowledge (30), and the links between concepts and fields of knowledge (though with plenty of caveats) (31). GPT3 is creating and synthesising text, and there are tools for encouraging creative combinations – such as Project Solvent (32) training algorithms to find analogies (33). The best of these combine collective intelligence and artificial intelligence. The same is true of the explosion of methods to help groups think together and achieve consensus – Miroboards for meetings and the AI Polis programme used in politics (34) – which aim to synthesise positions and counter the social media propensity to amplify differences.
Syntheses can close down or open up
Synthesis processes and outputs can either open up intelligence and action or close it down. The Soviet Union’s attempt at comprehensive planning, with brilliant mathematicians attempting to optimise economic operations, was in retrospect closing and flattening operations – unable to cope with unpredictable change or to prompt innovation. In the same way, some cost benefit analyses, and some of the more extreme claims for ‘implementation science’, block off debate rather than encouraging it (and are subject to many biases and errors).
Better syntheses allow a continuing process of improvement, with rich feedback that can be used to challenge and adjust the synthesis, identifying key indicators to be tracked. This is set to be particularly important in the wave of inquiries into COVID-19 that are set to begin in 2022. These can either aim to close down debate with simple conclusions on who is to blame, or they can be used to spark more reflecting learning.
In my recent paper on wisdom (35), I suggested we should think about synthesis of multiple elements of intelligence as a kind of loop, best cultivated through explicit prediction, observation and then updating or adaptation of models. The paper shows how this simple idea can be applied to institutions, technologies and individual lives.
What might be done?
In what we hope are the later stages of the COVID-19 pandemic, what might be done to address the imbalances between advice and absorption, analysis and synthesis? One implication of this paper is that organisations devoted to knowledge should give more priority to methods for synthesis.
Specifically, universities have lost sight of their role as integrators, synthesisers and promoters of wisdom, favouring instead disciplinary knowledge. Courses that were once pioneers of new methods of synthesis – such as PPE at Oxford, invented a century ago – now look ill-suited to the kinds of knowledge needed by decision-makers.
Meanwhile, I would argue that the many good programmes designed for knowledge synthesis or knowledge brokering need to be complemented by more explicit attention to the kinds of synthesis for action described in this paper. For centres of government, the implication is that they should prioritise better synthesis on at least three fronts:
- First, encouraging skills for senior decision-makers that fit the likely patterns they will face (i.e. knowledge of science, engineering, logistics, data, psychology as well as the law and economics, which now tend to be well-represented in senior levels of government) to enable them to integrate complex information, and explicit training in the steps set out earlier. Howard Gardner’s ideas on how to cultivate the ‘synthesising mind’ also provide useful options. (36)
- Second, structures that do not simply multiply advisory inputs and committees, but rather complement these with specialist integrators and synthesisers, organised in multi-disciplinary teams that can rapidly apply the kind of processes described above.
- Third, promoting explicit methods for integration and synthesis, along the lines described earlier, that can be refined and improved over time using feedback loops to improve capability – i.e. learning lessons in an open and rigorous way to update models, and using the array of technologies now available to help teams do their work. A critical role for such methods is to create a common language – it’s very hard to synthesise when every discipline uses different jargon.
A conclusion: the various synthesis gaps
This paper argues that there are serious ‘synthesis gaps’ that need to be addressed – in the workings of governments and also of universities and other organisations charged with mobilising and using knowledge. It argues that these gaps can be addressed through a more conscious focus on the skills, structures and processes needed for synthesis and integration.
It was prompted by a widespread perception that many governments lack good mechanisms for synthesis – with advice fed into central units or ministers without adequate integration or synthesis – and that this could be one of many factors that has led to bad decisions.
It was also prompted by a sense that there is a parallel ‘synthesis gap’ in writings on artificial intelligence and computer science. Algorithms are used to make or guide many decisions (from mortgages to diagnoses), but most require at least some human input to handle less usual cases, and all difficult decisions require some combination of incommensurable elements that go far beyond the capability of any AI.
I hope the paper can prompt better understanding of how synthesis is organised – and sharper thinking about how it is done well, whose job it is, and how to do it better.
Author’s note
Thanks to Sir Peter Gluckman, Scott Page, Kristiann Allen, Adam Cooper, Eirini Malliariki, Mike Herd and other colleagues at IPPO and UCL STEaPP for their comments on an earlier draft of this paper.
Footnotes
(1) Integration means combining parts to make a whole – its meanings overlap with synthesis though with a Latin rather than Greek etymology.
(2) I’m aware that many would argue that governments don’t do this: they improvise or follow intuitions. But it’s hard to imagine any ideal of good government that doesn’t require some formal synthesis.
(3) https://www.nature.com/articles/d41586-018-05414-4
(4) There may also be glaring gaps – as the UK found with the weakness of data and feedback from the care system, mental health and community compliance, or knowledge of how to counter social media misinformation.
(5) See, for example, the recent report from the European Commission JRC on values: https://ec.europa.eu/jrc/en/news/joint-research-centre-s-new-report-calls-systematic-consideration-values-and-identities-eu; and the recent report from Peter Gluckman and Anne Bardsley on high impact risks: https://informedfutures.org/wp-content/uploads/High-impact-risks.pdf
(6) E.g. through the ‘Basic Human Values’ theory of Shalom Schwartz and the theory of ‘materialist’ and ‘post-materialist’ models used by Ronald Inglehart and the World Values Survey, both of which have generated huge amounts of data and analysis on patterns of values across the world.
(7) Gluckman, Bardsley and Kaiser, 2021: https://www.nature.com/articles/s41599-021-00756-3
(8) Its Greek origins link the word analysis to ideas of breaking up, unloosing, releasing and setting free.
(9) Synthesis comes from ancient Greek σύνθεσις, which combines σύν (with) and θεσις (placing). It means any kind of integration that brings together more than one element and creates something new. It happens in cooking, music, architecture, editing, poetry, detective work, negotiation and democracy. It is part of how nature works – creating new chemical compounds, cells and organisms.
(10) Critique of Pure Reason, Immanuel Kant
(11) Another metaphor is energy: how two conflicting or opposing pressures can come together into a shared forward movement.
(12) Coleridge was borrowing from Friedrich Schelling’s concept of ‘einbildungskraft’, which formed part of a grand theory of imagination. Gary Lachman, Lost Knowledge of the Imagination.
(13) John Dewey, Late Works, 10:271
(14) I’m grateful to Scott Page for suggesting this distinction.
(15) I explain this point in more detail in my book ‘Big Mind’, p 67 and following.
(16) My book ‘The Art of Public Strategy’ sets out what this means at much greater length, and why understanding limitations of power and knowledge is so essential for understanding any issues of public policy.
(17) There are huge numbers of options with a sea of acronyms contributing to EBPM: MCA, CRELE, ACTA and others.
(18) For a useful account of the difference between linear and iterative models, see the guidance note from the UNDESA Committee of Experts on Public Policy on Science Policy Interface (written by K. Allen): Strategy note: science policy interface, March 2021.pdf (un.org)
(19) As documented in detail in Ian McGilchrist’s work on the left and right brain, including the latest, ‘The Matter with Things’.
(20) NICE includes a formal process for engaging with the perspectives of different stakeholders to define the research questions and thus the evidence considered, how it is synthesised, and how it is interpreted. See Gough (2021): Appraising evidence statements https://doi.org/10.3102/0091732X20985072
(21) I recently wrote this piece on some of the options for inquiries. IPPO also shared this broader overview of the issues and options.
(22) See Scott Page’s book ‘Model Thinking’ for a brilliant account of the virtues of using multiple models to grasp complex phenomena.
(23) Recent initiatives such as the ARIs and the changed status of Chief Scientific Advisers are a modest step in the direction indicated. The bigger barriers however are more structural – the lack of institutions with the capability and authority to do the work of integration and synthesis. This paper set out ideas on how the centre of a government should be organised:
(24) Though it is still surprisingly rare in universities, which tend to favour deepening within disciplines rather than combinations, and it is often difficult within governments which prefer more linear approaches to problem-solving.
(25) Scott Page, Diversity and Complexity. Princeton (NJ): Princeton University Press, 2011.
(26) See, for example, much of the work by Philip Tetlock over the last 20 years.
(27) Economics has at times aspired to become a meta-language too, and has had some success in encouraging other disciplines to adapt (for example with parts of psychology rebranding themselves as behavioural economics).
(28) To bridge ‘structural holes’, in the language of Ronald Burt.
(29) Boris Johnson’s adviser Dominic Cummings for a time advocated bringing more cognitive diversity into the heart of government, but missed other crucial parts of this story, including practical frontline knowledge (covered in my book ‘The Art of Public Strategy’, Oxford University Press, 2008). One of the odd patterns of recent years has been a deterioration in the quality of strategy work in many governments – partly an effect of shorter time horizons and changing political culture, particularly since the financial crisis of 2007/8. The result in at least some governments has been a serious reduction in anticipatory capacity – such as the ability to anticipate the effects of a pandemic or Brexit.
(30) Thanks to Eirini Malliariki and Aleks Berditchevskaia for their interesting recent piece on ‘The future of human and machine intelligence at the knowledge frontier’. Other relevant references in this space include: Open Research Knowledge Graph, DBpedia, Eccenca, Wolfram Alpha, Wikidata, Yago, Open Targets, Scibite, BioRelate, EBI’s Expression Atlas, SATORI TreeMap; Auer, S., Kovtun, V., Prinz, M., Kasprzik, A., Stocker, M. and Vidal, M.E., 2018, June. Towards a knowledge graph for science; Proceedings of the 8th International Conference on Web Intelligence, Mining and Semantics (pp. 1-6). Chaudhri, V.K., Chittar, N. and Genesereth, M. 2021. What is a Knowledge Graph? Stanford.
(31) https://dongshengwang.medium.com/5-reasons-knowledge-graph-will-never-bloom-418601957f33
(32) Chan, J., Chang, J.C., Hope, T., Shahaf, D. and Kittur, A., 2018. Solvent: A mixed initiative system for finding analogies between research papers. Proceedings of the ACM on Human-Computer Interaction, 2(CSCW), pp.1-21.
(33) Hope, T., Tamari, R., Kang, H., Hershcovich, D., Chan, J., Kittur, A. and Shahaf, D., 2021. Scaling Creative Inspiration with Fine-Grained Functional Facets of Product Ideas. arXiv preprint arXiv:2102.09761.
(34) E.g. in the Taiwanese parliament’s vTaiwan and other projects. Polis and equivalents are not yet used in UK politics, as far as I am aware.
(35) Geoff Mulgan, Wisdom as a Loop, Demos Helsinki, 2021: https://demoshelsinki.fi/julkaisut/loops-for-wisdom-cultivate-wisdom-in-society/